The Mediating Role of Loneliness on the Relationship Between Internet Addiction and Burnout Levels: A PLS-SEM Approach
Abstract:
Purpose: The aim of this study is to examine the mediating role of loneliness between the internet addictions of accountants with their burnout levels. Methodology: Using the survey method, 231 responses were solicited from accountants in Turkiye. A partial least squares structural equation model was constructed in order to test both the reliability and the validity of the measurement, as well as the structural model. Findings: The results indicated that loneliness partially mediated the relationship between internet addiction with emotional exhaustion and depersonalization, and reduced personal accomplishment levels of burnout. Originality/Value: This empirical study investigates the mediating role of loneliness between internet addiction and burnout levels among accountants, using the Partial Least Squares (PLS) regression analysis approach.
1. Introduction
Burnout has long been a common phenomenon among accountants (Herda and Lavelle, 2012). The accounting profession has been connected to high turnover rates (Fogarty and Uliss 2000); it has always been highly stressful. Demanding deadlines, limited time for personal life, and the high risk of possible firings creates stress for accountants, leading to emotional exhaustion, and resulting in a tendency toward higher turnover intentions (Jones et al., 2010; Chong and Monroe, 2015). Considering the serious consequences of burnout for both accountants and accounting firms, it is crucial to study possible remedies with which to prevent it. In order to prevent and mitigate the burnout experienced by accountants, it is very important to understand it’s determinants (Herda and Lavelle, 2012; Lambert et al., 2013). While there are a lot of studies which have examined job-related stressors as determinants of burnout, the literature focusing on the relationship between internet addiction and burnout among accountants is severely limited.
Internet addiction, which is characterized by excessive and uncontrollable internet usage, has been identified as a severe problem, especially among the younger population (Young, 1998). Several studies have indicated that excessive use of the internet also creates potentially harmful effects, such as poor grade performance of students, discord within couples, and decreased work performance among employees (Young, 1998). Further research has revealed that internet addiction is a significant predictor of burnout (Liu and Ma, 2020). On the other hand, the combination of internet addiction with loneliness have been frequently examined together (Lelkes, 2013). Loneliness is defined as an unwelcome perceived feeling of social isolation (Beneito-Montagut, Cassián-Yde, and Begueria, 2018). A number of studies have shown the relationship connecting internet addiction with loneliness and burnout (Kraut et al., 1998). Although several studies have focused on the relationship between internet addiction, loneliness, and burnout, there is a scarcity of research on the mediating role of loneliness between internet addiction and burnout levels. Also lacking are empirical studies investigating these relationships within the ranks of accountants. Therefore, the purpose of this study is to examine the mediating role of loneliness within the relationship between internet addiction and burnout levels experienced by accountants.
Burnout has been described as ‘a state of exhaustion that results from failure, attrition, loss of energy and power, or unfulfilled wishes on human internal resources’ (Freudenberger, 1974, p. 160). In the last decade, much research concerning burnout has highlighted different business areas. In one of the most widely accepted conceptualizations, it has been described as being composed of three dimensions, being: emotional exhaustion, depersonalization, and reduced personal accomplishment (Maslach and Jackson, 1986). While emotional exhaustion refers to the depletion of emotional and physical resources wherein the individual lacks the necessary energy to perform the required work, depersonalization is an uncaring and negative attitude toward different aspects of the job. Reduced personal accomplishment imply feelings of incompetency, lack of achievement, and low work productivity.
Reports in the literature state that the main causes of job burnout are workplace factors, rather than the personal characteristics of employees (Maslach and Jackson, 1981). It has also been observed that employees working in stressful jobs and/or workplaces are likely to suffer burnout (Maslach et al., 2001; Marek et al., 2017). Accountants have been ranked among the top ten most stressful occupations in the USA (Miller et al., 1988). Burned out accountants manifest a high turnover intention, poor job performance, and experience low levels of job satisfaction (Jones et al., 2010; Chong and Monroe, 2015). Although many studies have focused on the determinants of burnout such as job stressors (e.g., role conflict or role ambiguity) (Kalbers and Fogarty, 2005) and job attitudes (Dinc et al., 2020), there is a scarcity of research on the relationship between internet addiction and burnout within the ranks of accountants.
Internet addiction is defined as ‘a psychological dependence on the internet, regardless of the type of activity once logged on’ (Kandell, 1998, p. 12). In the short term, internet addiction can be characterized by excessive and uncontrollable internet use (Young, 1998). The term ‘excessive use’, which appears in many studies of internet addiction, usually indicates that the amount of time spent online is an important factor for determining internet addiction. In Young’s study (1998), internet dependents reported spending a striking average of 39 hours per week online for nonacademic or nonemployment purposes, compared to the 5 hours reported by those who were not addicted to the internet. In recent years, this addiction has been identified as a severe problem, especially among the young population (Young, 1998) since it causes harmful effects such as poor grade performance among students, disagreement between partners, and diminished work performance among employees. Further research has indicated that significant levels of depression are associated with pathological internet use (Young and Rogers, 1998; Seabrook et al., 2016). For example, a study conducted with 93 families indicated that greater internet usage was positively linked to daily stress, depression, and loneliness. (Kraut et al., 1998).
Loneliness, which has been conceptualized under the terms of ‘perceived social isolation’ (Cacioppo et al. 2009) and ‘emotional isolation’ (Ditommaso et al., 2004), is defined as a ‘discrepancy between one’s desired and achieved levels of social relations’ (Peplau and Perlman 1982, 32). It is a discrepancy that is experienced between the type of personal relationships that individuals have with the relationships they would like to have (Sermat, 1978). So, loneliness can be understood as the opposite of “social support” and a lack of company, and it is mostly related to the quality of the relationships a person enjoys (Grenade and Boldy 2008). Previous studies have shown that there is a positive relationship between internet use and loneliness (Lelkes, 2013; Beneito-Montagut, Cassián-Yde, and Begueria, 2018). In other words, those who are already lonely have a tendency to use the internet frequently (Tokunaga and Rains, 2010). However, studies provide strong evidence of the harmful effects through loneliness on health and wellbeing (Lunstad et al. 2015, Miyawaki, 2015). On the other hand, the literature has shown that frequency of internet use has been associated with loneliness and depression (Kraut et al., 1998). Based on the previous literature discussed above, the following hypotheses are posited:
Hypothesis 1: Loneliness mediates the relationship between Internet Addiction and Emotional Exhaustion
Hypothesis 2: Loneliness mediates the relationship between Internet Addiction and Depersonalization
Hypothesis 3: Loneliness mediates the relationship between Internet Addiction and Personal Accomplishment
2. Research Methodology
The research hypotheses are tested using PLS-SEM (Partial Least Square based Structural Equation Modeling). As well, mediation analysis is utilized using Preacher & Hayes’s (2008) methodology with the PROCESS software. The research model is shown in Figure-1 below. It indicates the direct relationship between the constructs as well as the indirect effects between internet addiction with personal accomplishment, emotional exhaustion, and depersonalization through loneliness.

Data Collection
The items of the constructs of the internet addiction were adapted Young (1996)’s scale.
The items of the constructs for loneliness were adapted from the UCLA-loneliness scale developed by Russell (1996).
The items of the constructs relating to emotional exhaustion, depersonalization, and personal accomplishment were adapted from the Maslach Burnout Inventory, developed by Maslach and Jackson (1981), which contained three components: “emotional exhaustion,” “depersonalization”, and “personal accomplishments”.
The scales are supplied in English. Therefore, the survey questions which are in the English language are translated into the Turkish language. We use Brislin’s (1966) back-translation method. The items in the constructs are measured using a five-point Likert Scale for which one indicates strongly disagree while five indicates strongly agree. The final form of the survey was then distributed.
The survey questionnaire was sent to respondents via an online survey tool. The participants were required to answer every question, thus ensuring that there is no missing values in the research sample.
3. Sample
After collection of the data set, data preprocessing was employed. There were no missing values, so no imputation was required. The variable inflation factors-VIF values show that there is no multicollinearity issue. The VIF values are all less than the cut-off value of ten (Hair et. al., 2010). The final sample size comprised 231 accountants.
The summary statistics based on demographic indicators are provided in Table 1. The results reveal that 45% of the participants are born between 1966 and 1979, while less than 2% are born after 1996. In addition, the results show that approximately 77% of the participants are male, and 23% are female, 79% are married, 16% are divorced, less than 2% have a Ph.D. degree, 73% have an undergraduate degree, almost 21% have more than 26 years of experience, and approximately 11% have less than 5 years ofexperience.
Variables | Categories | Frequency | Percent |
Age level (Born year) | 1945-1965 | 40 | 17.30 |
| 1966-1979 | 104 | 45.00 |
| 1980-1995 | 84 | 36.40 |
| 1996- | 3 | 1.30 |
| Total | 231 | 100.00 |
Gender (Male vs Female) | Male | 177 | 76.60 |
| Female | 54 | 23.40 |
| Total | 231 | 100.00 |
Marital status (Single, divorced, & married) | Single | 37 | 16.02 |
| Divorced | 11 | 4.76 |
| Married | 183 | 79.22 |
| Total | 231 | 100.00 |
Education level (High school – Ph.D. level) | Ph.D. | 3 | 1.30 |
| Master | 41 | 17.70 |
| Undergraduate | 169 | 73.20 |
| Associate | 9 | 3.90 |
| High School | 9 | 3.90 |
| Total | 231 | 100.00 |
Experience (Years) | 1-5 | 26 | 11.30 |
| 6-10 | 32 | 13.90 |
| 11-15 | 47 | 20.30 |
| 16-20 | 37 | 16.00 |
| 21-25 | 40 | 17.30 |
| 26+ | 49 | 21.20 |
| Total | 231 | 100.00 |
4. Factor Analysis
The items of the constructs are subject to EFA (Exploratory Factor Analysis) even though the scales of the constructs were adapted or modified in accordance with the aforementioned literature. The PAF (Principal Axis Factoring) as the extraction method , and the Promax as the rotation method are used to find the factors. The EFA’s results are shown in Table 2. These results indicated that 39 items remained, following the elimination of the items with low factor loadings: ten items for internet addiction, sixteen items for loneliness, five items for emotional exhaustion, four items for depersonalization, and four items for personal accomplishment are used for measuring the indicated constructs. The factor loadings for internet addiction ranged between .62 and .81, for loneliness ranged between .60 and .85, for emotional exhaustion ranged between .71 and .89, for depersonalization ranged between .75 and .89, and finally, for personal accomplishment, ranged between .68 and .78, all of which are above the minimum factor loading of .35 based on the sample size of 231 (Hair, et. al., 2010). Moreover, the portion of the total variance that accounted for the extracted factors ranged between 2.38% (personal accomplishment) and 30.96% (internet addiction) while the cumulative portion of variance for which all the factors were accounted was 54.12%. In addition, the eigen-values of the latent variables ranged between 1.79 and 7.90 (based on after rotation). Furthermore, the adequacy of the used sample data set for EFA was satisfied, based on KMO (Kaiser, Meyer, & Olkin) test statistics (KMO = 0.91), which is greater than the suggested cut-off value of .70 (Hair et. al., 2010). Moreover, the Barlett’s Sphericity test show that variables are sufficiently related to each other to perform EFA (χ2=5706.54, df =741, p-value
=0.0001). Convergent validity, discriminant validity, and reliability were satisfied. The items within each factor are highly correlated, thus convergent validity is satisfied. Moreover, the items of the factors are distinct and uncorrelated. The items have high loadings under each factor with there is no significantly high cross loadings among factors. Thus, discriminant validity is satisfied. Finally, Cronbach’s Alpha results range between .72 and .94 which are greater than the suggested value of 0.70 (Hair et. al., 2010). Therefore, the reliability is satisfied.
Items | IAD | LON | EEX | DEP | PAC |
IAD1 | 0.72 | 0.21 | 0.18 | 0.23 | 0.09 |
IAD2 | 0.65 | 0.25 | 0.27 | 0.36 | -0.10 |
IAD3 | 0.81 | 0.30 | 0.24 | 0.32 | 0.03 |
IAD4 | 0.81 | 0.34 | 0.28 | 0.31 | -0.04 |
IAD5 | 0.71 | 0.27 | 0.29 | 0.35 | -0.04 |
IAD6 | 0.74 | 0.30 | 0.27 | 0.29 | -0.04 |
IAD7 | 0.68 | 0.30 | 0.24 | 0.28 | 0.01 |
IAD8 | 0.78 | 0.34 | 0.30 | 0.35 | -0.02 |
IAD9 | 0.63 | 0.33 | 0.35 | 0.39 | -0.06 |
IAD10 | 0.62 | 0.23 | 0.30 | 0.39 | 0.01 |
LON1 | 0.13 | 0.64 | 0.23 | 0.32 | -0.42 |
LON2 | 0.41 | 0.80 | 0.43 | 0.51 | -0.30 |
LON3 | 0.39 | 0.75 | 0.35 | 0.47 | -0.29 |
LON4 | 0.34 | 0.76 | 0.41 | 0.46 | -0.20 |
LON5 | 0.42 | 0.85 | 0.45 | 0.53 | -0.25 |
LON6 | 0.07 | 0.60 | 0.17 | 0.22 | -0.43 |
LON7 | 0.18 | 0.70 | 0.29 | 0.36 | -0.43 |
LON8 | 0.32 | 0.69 | 0.43 | 0.40 | -0.34 |
LON9 | 0.41 | 0.71 | 0.45 | 0.45 | -0.18 |
LON10 | 0.10 | 0.72 | 0.21 | 0.27 | -0.43 |
LON11 | 0.12 | 0.68 | 0.16 | 0.21 | -0.40 |
LON12 | 0.37 | 0.69 | 0.37 | 0.39 | -0.13 |
LON13 | 0.44 | 0.80 | 0.53 | 0.48 | -0.29 |
LON14 | 0.09 | 0.61 | 0.21 | 0.25 | -0.37 |
LON15 | 0.09 | 0.63 | 0.19 | 0.26 | -0.42 |
LON16 | 0.37 | 0.78 | 0.41 | 0.45 | -0.23 |
EEX1 | 0.33 | 0.41 | 0.85 | 0.51 | -0.15 |
EEX2 | 0.34 | 0.43 | 0.88 | 0.61 | -0.09 |
EEX3 | 0.33 | 0.39 | 0.89 | 0.61 | -0.12 |
EEX4 | 0.33 | 0.47 | 0.88 | 0.57 | -0.14 |
EEX5 | 0.30 | 0.36 | 0.71 | 0.45 | -0.05 |
DEP1 | 0.32 | 0.37 | 0.46 | 0.78 | -0.04 |
DEP2 | 0.40 | 0.49 | 0.59 | 0.89 | -0.13 |
DEP3 | 0.38 | 0.45 | 0.65 | 0.85 | -0.06 |
DEP4 | 0.38 | 0.46 | 0.42 | 0.75 | -0.10 |
PAC1 | 0.03 | -0.25 | -0.14 | -0.01 | 0.70 |
PAC2 | 0.03 | -0.38 | -0.06 | -0.11 | 0.78 |
PAC3 | -0.12 | -0.26 | -0.11 | -0.07 | 0.68 |
PAC4 | -0.04 | -0.32 | -0.10 | -0.10 | 0.77 |
Alpha* | 0.890 | 0.940 | 0.900 | 0.83 | 0.72 |
Variance (%) | 30.96 | 11.32 | 6.23 | 3.24 | 2.38 |
Cumulative percent of variance | 30.96 | 42.28 | 48.51 | 51.75 | 54.12 |
Eigenvalues after rotation | 7.90 | 7.37 | 8.41 | 9.81 | 1.79 |
Confirmatory Factor Analysis-CFA: The extracted five constructs are examined using confirmatory factor analysis. Thus, the latent variables (five factors) in a single model are included in CFA to determine their reliability, validity, and constructs’ the model-fits (Fornell and Larcker, 1981). Table 3 reports the standardized regression weights, the values of the t-statistics of the corresponding items under each construct, and the model-fit performance measures. The model-fit measures indicate how well the factor structure accounts for the association between the variables in the dataset. The ML-Maximum Likelihood estimator is employed for the CFA. The analysis results, based on the ML estimator, indicated that χ2/df is 1.71, the CFI (comparative fit index) is 0.92, the IFI (incremental fit index) is 0.93, the TLI (Tucker-Lewis index) is 0.91, the RFI (relative fit index) is 0.90, the GFI (goodness of fit index) is 0.90, and the RMSEA (root mean square error of approximation) is 0.05, all of which are sufficient based on the recommended threshold values (Bagozzi &Yi, 1988, and Hu & Bentler, 1999). As a result of the model-fit measurements, which were based on confirmatory factor analysis, a well model-fit measures are obtained.
Latent variable | Items | Standardized Regression Weights | t-value |
Internet Addiction | IDA1 | 0.74 | Scaling |
IDA2 | 0.60 | 7.39 | |
IDA3 | 0.75 | 11.14 | |
IDA4 | 0.79 | 10.38 | |
IDA5 | 0.70 | 9.01 | |
IDA6 | 0.70 | 10.03 | |
IDA7 | 0.62 | 8058 | |
IDA8 | 0.72 | 9079 | |
IDA9 | 0.56 | 7.28 | |
IDA10 | 0.59 | 8.13 | |
Loneliness | LON1 | 0.75 | Scaling |
LON2 | 0.70 | 10.71 | |
LON3 | 0.67 | 9.36 | |
LON4 | 0.71 | 10.57 | |
LON5 | 0.77 | 12.84 | |
LON6 | 0.62 | 9.01 | |
LON7 | 0.73 | 10.40 | |
LON8 | 0.67 | 10.16 | |
LON9 | 0.67 | 10.12 | |
LON10 | 0.70 | 10.51 | |
LON11 | 0.64 | 9.59 | |
LON12 | 0.65 | 9.29 | |
LON13 | 0.79 | 11.91 | |
LON14 | 0.58 | 8.42 | |
LON15 | 0.59 | 8.62 | |
LON16 | 0.73 | 10.58 | |
Emotional Exhaustion | EEX1 | 0.79 | Scaling |
EEX2 | 0.86 | 11.77 | |
EEX3 | 0.88 | 12.58 | |
EEX4 | 0.84 | 11.51 | |
EEX5 | 0.61 | 9.69 | |
Depersonalization | DEP1 | 0.68 | Scaling |
DEP2 | 0.80 | 9.68 | |
DEP3 | 0.80 | 9.96 | |
DEP4 | 0.61 | 7.89 | |
Personal Accomplishment | PAC1 | 0.66 | Scaling |
PAC2 | 0.55 | 6.23 | |
PAC3 | 0.68 | 6.70 | |
PAC4 | 0.64 | 6.38 |
Measurement Model: Prior to testing the proposed hypothesis using Structural Equation Modeling (SEM), an investigation into the validity, reliability, and consistency of the latent variables was crucial (Hair, et. al., 2010). The Correlation analysis based on Pearson’s coefficients, summary statistics, Average Variance Extracted (AVE) values, Composite Reliability (CR), Cronbach’s Alpha and the square root of AVE values on the diagonal of the correlation matrix are provided in Table 4. The analysis results indicated that the reliability of the constructs was satisfactory, since the corresponding values of the latent variables’ reliability values based on Cronbach’s Alpha (ranging between 0.72 and 0.94) and the CR (ranging between 0.82 and 0.94) are both higher than the suggested value of 0.70 (Nunnally and Bernstein, 1994). Moreover, the convergent validity is satisfied since the AVE values (ranging between 0.51 and 0.72) are fairly above the suggested value of .50. Finally, the discriminant validity is satisfied since the square root of the AVE records at the diagonal of the matrix are greater than any inter-correlation coefficients.
Latent Variables | L1 | L2 | L3 | L4 | L5 |
(1) Internet addiction | .72 |
|
|
|
|
(2) Loneliness | .30** | .73 |
|
|
|
(3) Emotional Exhaustion | .39** | .45** | .85 |
|
|
(4) Depersonalization | .45** | .51** | .65** | .82 |
|
(5) Personal accomplishment | .05 | -.44** | -0.12 | -.09 | .73 |
Mean | 2.00 | 2.53 | 3.09 | 2.56 | 3.62 |
Std. Deviation | .72 | .81 | 1.16 | 1.05 | .74 |
Average Variance Extracted (AVE) | .51 | .53 | .72 | .67 | .54 |
Composite Reliability | .91 | .94 | .93 | .89 | .82 |
Cronbach’s Alpha | .89 | .94 | .90 | .83 | .72 |
Mediation Analysis: The hypotheses regarding mediation roles of selected variable are tested. The corresponding results are shown in Table 6, 7, 8, and 9. They summarize the association of internet with emotional exhaustion, depersonalization, and personal accomplishment via loneliness. For this analysis, the PROCESS Macro is used (Hayes, 2013) where the Bias-Corrected Bootstrapping methodology was used.
Bootstrapping for the calculations of the 95% bias-corrected CIs (confidence intervals) of the direct, as well as the indirect, effects included the recommended number of 5,000 bootstrap resampling (Preacher and Hayes, 2008). In mediation analysis results, the effects obtained are statistically significant when the corresponding CI does not include zero. First of all, the mediation occurs when the indirect effect is statistically significant, namely the confidence interval does not include a zero value. In addition, the partial or the full mediation is determined by the significance level of direct effect. A full mediation occurs when the direct effect is not statistically significant, given that the indirect effect is significant, while a partial mediation occurs when the direct effect is statistically significant, given that the indirect effect is significant.
The mediation analysis results revealed that the indirect effect between internet addiction and emotional exhaustion, depersonalization, and personal accomplishment through loneliness were all statistically significant since the corresponding confidence intervals did not include zero, which confirms that mediation occurred. The next step was to determine whether these mediations were full or partial, following which the direct effects were investigated. Based on the significance levels of the direct effects, the mediations were all partial, since once again, the confidence levels of the direct effects of the proposed hypothesis did not include a zero value. Based on the mediation analysis results, there was a partial mediation between internet addiction with emotional exhaustion, depersonalization, and personal accomplishment through loneliness. Thus, the proposed hypotheses H1, H2, and H3 weresupported.
Hypothesis | Direct Effect | Indirect Effect | Result |
H1) Internet Addiction→ Loneliness→ Emotional Exhaustion | 0.45 | 0.18 | Partial mediation |
LLCI=.26, ULCI=.64 | LLCI=.09 ULCI=.29 | ||
H2) Internet Addiction → Loneliness → Depersonalization | 0.48 | 0.18 | Partial mediation |
LLCI=.32 ULCI=.64 | LLCI=.11 ULCI=.28 | ||
H3) Internet Addiction → Loneliness → Personal Accomplishment | 0.20 | -0.15 | Partial mediation |
| LLCI=.08 ULCI=.32 | LLCI= -.24 ULCI=-.09 |
|
Baron and Kenny (1986)’s four steps are shown below. The baseline analysis which incorporates the methodology of the four steps. The independent variable is denoted as (X) which is “human capital investment”; the mediator variable is denoted as (M) which is “innovation capital”, and the dependent variables is denoted as (Y) are which are “ROA” and “Tobin’s Q”.
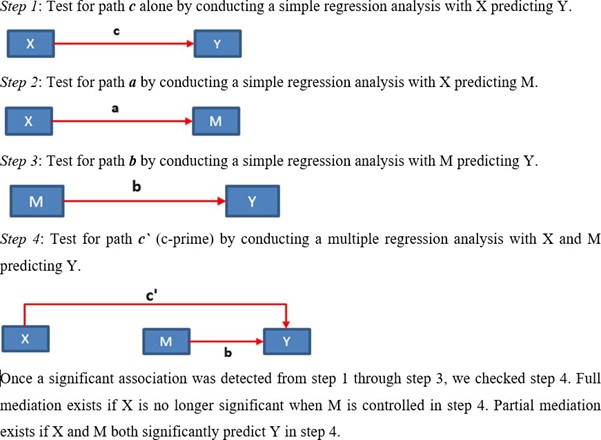
| (1) | (2) | (3) | (4) |
| Emotional Exhaustion | Loneliness | Emotional Exhaustion | Emotional Exhaustion |
| Step-1 | Step-2 | Step-3 | Step-4 |
Internet Addiction | 0.63*** | 0.35*** |
| 0.44*** |
| (6.94) | (4.84) |
| (5.10) |
Loneliness |
|
| 0.66*** | 0.54*** |
|
|
| (8.84) | (7.31) |
Constant | 1.74*** | 1.81*** | 1.42*** | 0.76*** |
| (8.47) | (10.91) | (7.10) | (3.34) |
N | 231 | 231 | 231 | 231 |
R2 | 0.17 | 0.09 | 0.25 | 0.33 |
adj. R2 | 0.170 | 0.089 | 0.251 | 0.325 |
F-stat. | 48.20*** | 23.46*** | 78.17*** | 56.37*** |
| (1) | (2) | (3) | (4) |
| Step-1 | Step-2 | Step-3 | Step-4 |
| Depersonalization | Loneliness | Depersonalization | Depersonalization |
Internet Addiction | 0.70*** | 0.35*** |
| 0.51*** |
| (8.24) | (4.84) |
| (6.42) |
Loneliness |
|
| 0.69*** | 0.55*** |
|
|
| (9.66) | (8.02) |
Constant | 1.05*** | 1.81*** | 0.82*** | 0.057 |
| (5.41) | (10.91) | (4.30) | (0.27) |
N | 231 | 231 | 231 | 231 |
R2 | 0.23 | 0.09 | 0.29 | 0.40 |
adj. R2 | 0.225 | 0.089 | 0.287 | 0.393 |
F-stat. | 67.89*** | 23.46*** | 93.36*** | 75.49*** |
| (1) | (2) | (3) | (4) |
| Step-1 | Step-2 | Step-3 | Step-4 |
| Personal Accomplishment | Loneliness | Personal Accomplishment | Personal Accomplishment |
Internet Addiction | 0.58** | 0.35*** |
| 0.22*** |
| (7.85) | (4.84) |
| (3.55) |
Loneliness |
|
| -0.41*** | -0.47*** |
|
|
| (-7.69) | (-8.59) |
Constant | 3.41*** | 1.81*** | 4.58*** | 4.25*** |
| (21.86) | (10.91) | (32.23) | (25.38) |
N | 231 | 231 | 231 | 231 |
R2 | 0.15 | 0.09 | 0.21 | 0.25 |
adj. R2 | 0.149 | 0.089 | 0.202 | 0.240 |
F-stat. | 0.72*** | 23.46*** | 59.17*** | 37.37*** |
5. Discussion and Implications
This study is an empirical attempt through which to develop a theoretical framework for discovering the mediating effects of Loneliness on the relationship between Internet Addiction and Burnout dimensions such as Emotional Exhaustion, Depersonalization, and Reduced Personal Accomplishment among white-collar workers. Accountants in Turkiye were surveyed using an instrument comprised of empirically validated items (Young 1996, Russel 1996, and Maslach and Jackson 1981). Employing the 4-step approach recommended by Baron and Kenny (1986), we found Loneliness to be a mediating variable in the relationship between Internet Addiction and Burnout dimensions (see Tables 7, 8 and 9). More specifically, Loneliness was a partial mediator in explaining the relationship between the independent variable Internet Addiction and the dependent variables of Emotional Exhaustion, Depersonalization and Reduced Personal Accomplishment. Loneliness along with Internet Addiction could explain 32% of Emotional Exhaustion, 39% of Depersonalization and 24% of Reduced Personal Accomplishment.
Burnout has been a recurring issue for accountants. It is critical that organizations succeed in reducing their employees’ loneliness. In order to achieve this, employers may consider fostering connections among the accountants through support groups and socialization opportunities. Further study of the link between Loneliness and Internet Addiction is warranted to assist practicing accountants to thrive at their profession. Some research has also recommended that if organizations establish and develop policies to support and meet the needs of their employees, the emotional link between employees and their organizations will be strengthened. This will improve the performance of the employees and decrease any levels of burnout at the same time (Stohr et al., 1992). This study results recommend that accounting firms should make efforts to decrease the Loneliness level together with the Internet Addiction of their employees, in order to reduce their burnout level. Failing to do so could potentially contribute to an increased likelihood of emotional exhaustion, personal accomplishment and depersonalization of their employees.
6. Conclusion
Our study found that Loneliness is a mediating factor in understanding the relationship between Internet Addiction and Burnout dimensions. By demonstrating the partial mediating effects of Loneliness, we have advanced knowledge of the relationship between the Internet Addiction and Burnout dimensions. The findings of the study are consistent with the literature (Holt-Lunstad et al., 2015; Miyawaki, 2015; Shaw and Gant, 2004; Kraut et al., 1998). The previous literature found that Internet addiction is positively correlated with depression and loneliness (Kraut et al., 1998). If internet usage can be managed properly, it can decrease loneliness and burnout significantly (Shaw and Gant, 2004).
7. Limitation/Future Work
The study has a few limitations. The sample included only accounting professionals. The choice of accounting professionals for the sample is a double-edged sword. Since accounting work is inherently solitary, it was an attractive subject to survey. Generalization of the findings from this study to other professional settings can be established only after more studies that include sample subjects from other disciplines as well are completed.
